Understanding the Importance of a Data Labeling Platform for Modern Businesses
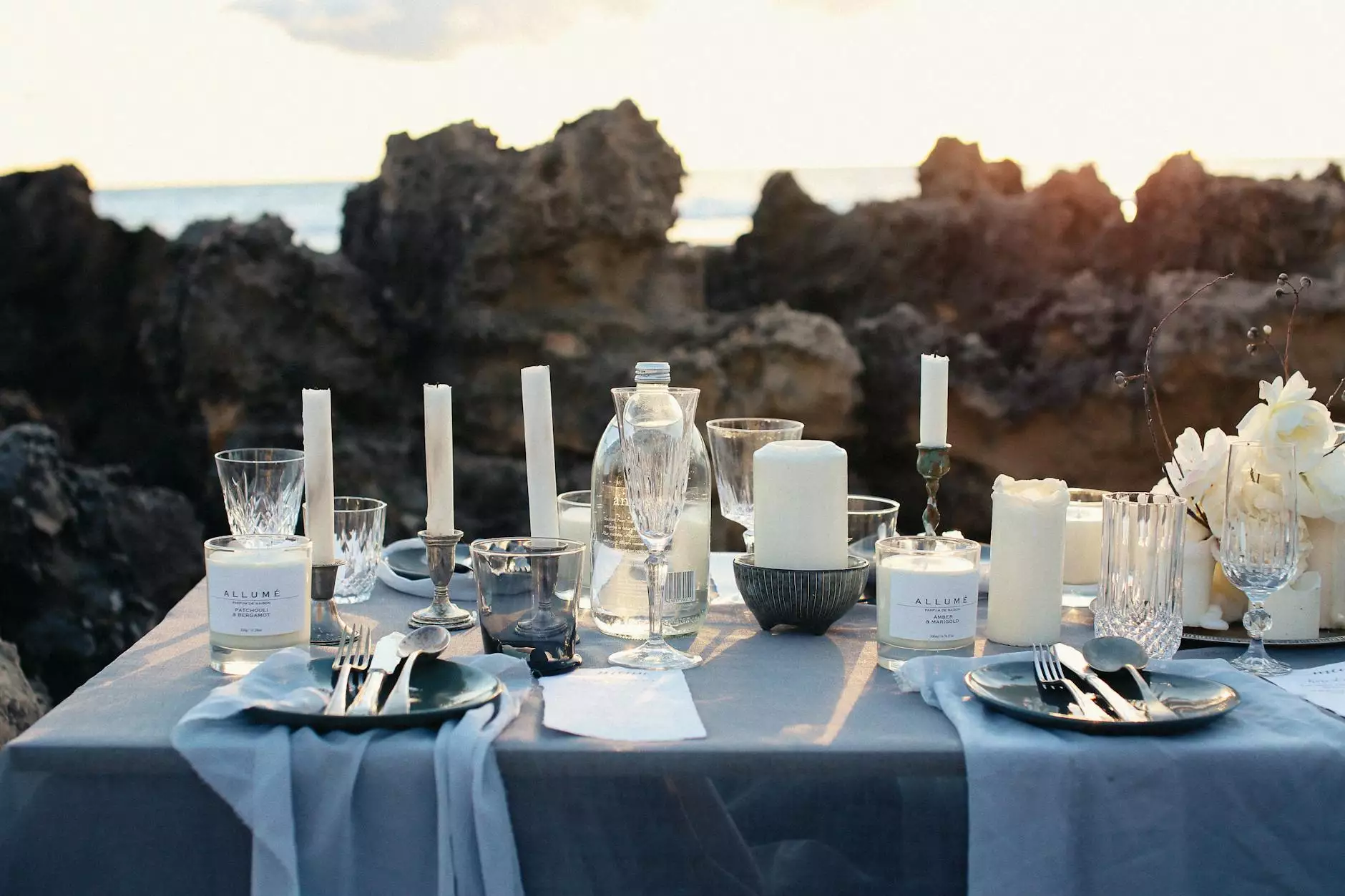
In today's rapidly evolving technological landscape, businesses require innovative solutions to harness the power of artificial intelligence (AI) and machine learning (ML). Data annotation, also known as labeling, has become a cornerstone of AI development, facilitating the training of algorithms through meticulous data preparation. A data labeling platform stands at the forefront of this endeavor, ensuring that businesses can meet their data needs efficiently and effectively.
What is a Data Labeling Platform?
A data labeling platform is a specialized software tool designed for annotating data sets. This platform provides functionalities to label various forms of data—such as text, images, audio, and video—making it an essential resource for companies looking to build machine learning models. By utilizing a data labeling platform, organizations can streamline their data preparation processes, increase accuracy, and ultimately improve the performance of their AI solutions.
Why Data Labeling is Critical for AI Development
Understanding the significance of data labeling is crucial for businesses aiming to implement AI technology. Here are a few key reasons why data labeling is essential:
- Quality Data: Machine learning models rely on high-quality, accurately labeled data to learn effectively. Poorly labeled data can lead to inaccurate predictions and unreliable results.
- Scalability: A well-structured data labeling platform enables businesses to scale their operations, accommodating larger data sets without compromising quality.
- Enhanced Efficiency: Automated data labeling tools reduce the time and effort required for manual annotation, allowing teams to focus on more complex tasks.
- Cost Effectiveness: By optimizing the labeling process, businesses can significantly reduce costs associated with data preparation.
Key Features of a Leading Data Labeling Platform
When choosing a data labeling platform for your business, it is essential to consider several key features that can enhance your experience and outcomes:
- User-Friendly Interface: An intuitive interface ensures that team members, regardless of technical proficiency, can engage with the platform efficiently.
- Support for Various Data Formats: The platform should accommodate a wide array of data types, including text, image, audio, and video, catering to diverse business needs.
- Collaboration Tools: Features that facilitate collaboration among team members can promote a more effective annotation workflow and improve communication.
- Customizable Workflows: The ability to create tailored workflows will enable businesses to meet specific project requirements without unnecessary complexity.
- Quality Control Mechanisms: Built-in quality assurance features help ensure that the labeled data meets rigorous standards, ultimately leading to better-performing models.
- Integration Capabilities: A robust platform should seamlessly integrate with other tools and systems used in the AI development process, such as data management and model training environments.
Benefits of Using a Data Labeling Platform
Implementing a data labeling platform provides numerous benefits that empower businesses on their AI journey. Below are some of the most significant advantages:
1. Accelerated Time to Market
Incorporating a data labeling platform can expedite the data preparation process, enabling businesses to launch AI-driven solutions faster than ever before.
2. Improved Model Performance
High-quality labeled data is crucial for training effective machine learning models. By utilizing a dedicated platform, businesses can enhance the accuracy and reliability of their models, leading to superior outcomes.
3. Enhanced Collaboration and Productivity
Data labeling platforms promote teamwork by providing collaborative tools, allowing teams to work together seamlessly and share feedback in real-time, thereby increasing productivity.
4. Access to Expert Annotation Services
Many platforms offer access to professional annotators who specialize in various domains. This means your data can be labeled by experts, ensuring higher accuracy and relevance for your specific use-case.
5. Continuous Learning and Improvement
Data labeling platforms often have built-in analytics that provide insights into the labeling process. This data can be used to refine workflows and improve future labeling tasks.
Choosing the Right Data Labeling Platform for Your Business
With numerous data labeling platforms available in the market, selecting the right one for your business can seem challenging. Here are some tips to guide your decision-making process:
- Assess Your Needs: Consider the types and volumes of data you need to label and ensure the platform you choose can meet those requirements.
- Read User Reviews: Feedback from existing users can provide insights into the platform’s ease-of-use, support, and effectiveness.
- Evaluate Cost: Look for a platform that provides excellent value for money without compromising on essential features.
- Test the Platform: Many providers offer free trials. Take advantage of these to evaluate the platform's capabilities and how well it fits your workflow.
Case Studies: Successful Implementations of Data Labeling Platforms
To illustrate the impact of data labeling platforms, let’s look at a few case studies:
1. Automotive Industry
A leading automotive manufacturer integrated a data labeling platform to enhance its autonomous vehicle recognition systems. By leveraging automated image annotations and expert oversight, the company reduced labeling time by 40% and significantly improved the accuracy of object detection algorithms.
2. E-commerce Sector
An e-commerce platform employed a data labeling solution to better understand customer behavior through sentiment analysis on product reviews. This insight allowed for targeted marketing strategies, ultimately leading to a 25% increase in sales conversions.
3. Healthcare Solutions
A healthcare technology firm used a data labeling platform to classify medical images for a diagnostic AI solution. The platform enabled precise annotations from domain experts, resulting in a model that achieved a two-fold increase in diagnostic accuracy compared to previous attempts.
Future Trends in Data Labeling
The data labeling platform landscape is poised for evolution as technology advances. Here are some emerging trends to watch:
1. Increasing Automation
As AI technology progresses, we can expect more sophisticated automation features in labeling platforms, reducing the need for manual input while maintaining accuracy.
2. Advanced Machine Learning Assistants
The incorporation of AI-driven assistants into labeling platforms will enhance the labeling process, providing real-time suggestions and automating repetitive tasks.
3. Enhanced Quality Control through AI
Future platforms may employ AI algorithms to assess the quality of labeled data dynamically, alerting teams to potential inaccuracies at an early stage.
4. Greater Focus on Collaboration
Expect to see a rise in platforms emphasizing collaborative features, enabling cross-functional teams to contribute to data labeling projects more effectively.
Conclusion
In conclusion, a data labeling platform is not just a tooling necessity—it is a strategic asset for any organization looking to capitalize on the power of AI and machine learning. By investing in a robust data labeling solution, businesses can ensure high-quality data, improve model accuracy, accelerate their time to market, and drive innovative solutions that meet the demands of today's fast-paced digital landscape.
As you explore options for integrating a data labeling platform into your operations, consider the aspects discussed in this article. With the right platform, you’ll be well on your way to transforming your business through enhanced data management and AI capabilities. Visit KeyLabs to learn more about the premier data annotation tools and platforms available to take your business further into the future of AI.