The Ultimate Guide to AI Labeling Tools
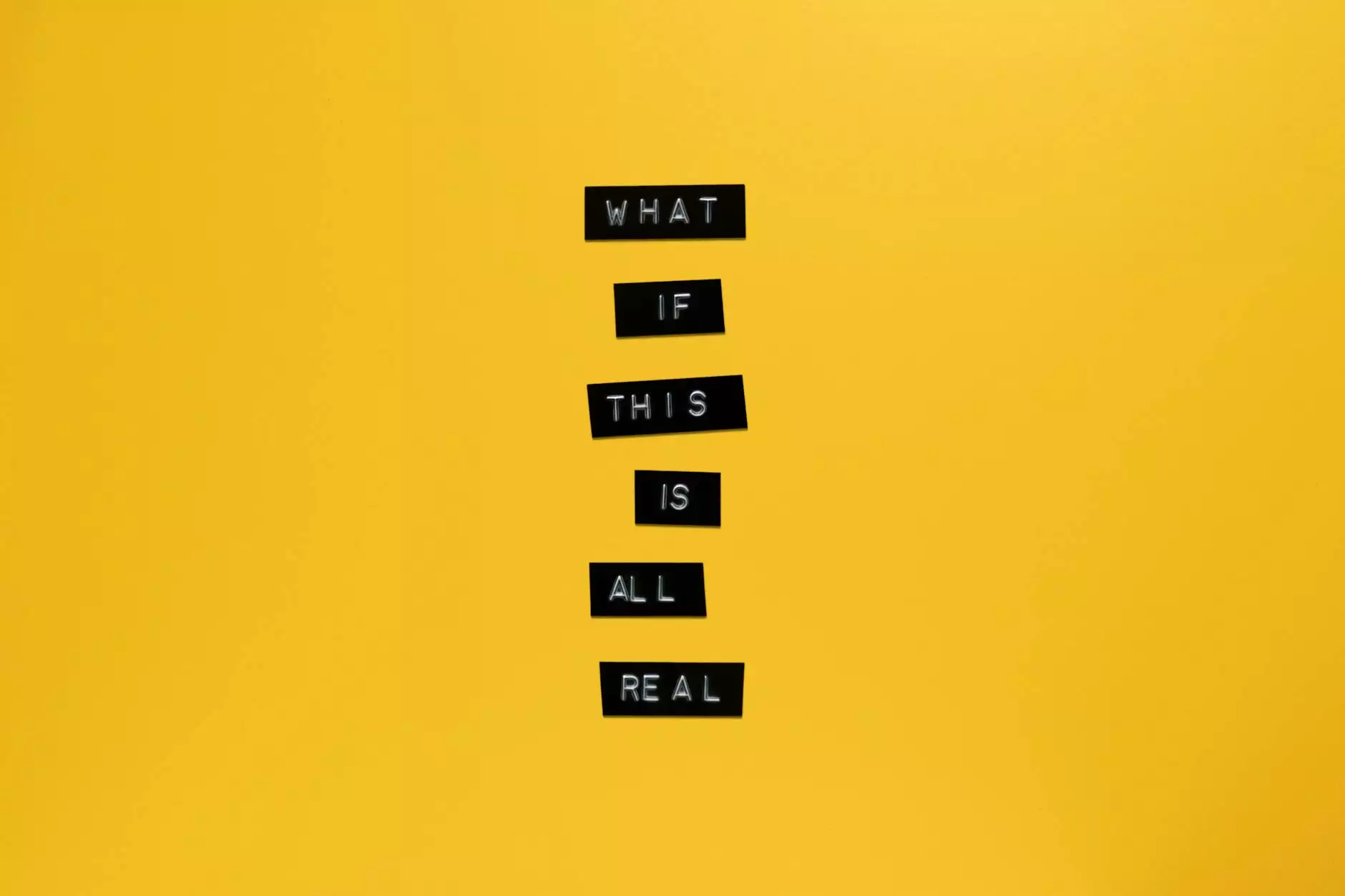
AI labeling tools are revolutionizing the way businesses approach data annotation. As companies increasingly rely on large datasets to train their artificial intelligence (AI) systems, the demand for accurate and efficient data labeling becomes paramount. In this comprehensive guide, we will explore the significance of AI labeling tools, the benefits they offer, their applications, and how key players like Keylabs.ai are leading the charge in data annotation solutions.
What Are AI Labeling Tools?
AI labeling tools are software applications designed to automate the process of annotating data. This process typically involves tagging or labeling various data types, such as images, text, and audio, to prepare them for machine learning algorithms. By using these tools, organizations can significantly reduce the time and costs associated with manual data annotation.
Types of Data Supported by AI Labeling Tools
- Image Data: Tools can annotate images by identifying and tagging objects, faces, or locations.
- Text Data: Textual data can be labeled with categories, sentiments, or keywords.
- Audio Data: Audio clips can be transcribed, tagged for speaker identification, or classified by emotional tone.
Why Use AI Labeling Tools?
The implementation of AI labeling tools provides several advantages for businesses seeking to leverage data more effectively. Here are some reasons why these tools have become essential:
1. Increased Efficiency
AI labeling tools can process large volumes of data in a fraction of the time it takes to do so manually. This time-saving capability allows companies to deploy AI models more rapidly.
2. Improved Accuracy
With machine learning algorithms continually evolving, AI labeling tools can provide more consistent and accurate annotations compared to human labelers, reducing errors and biases.
3. Cost-Effectiveness
By automating the data labeling process, organizations can significantly decrease labor costs associated with manual annotation efforts. Many businesses report substantial savings when integrating AI labeling tools into their workflows.
4. Scalability
As the amount of data increases, so too does the need for scalable labeling solutions. AI labeling tools can easily adapt to volume changes, accommodating a growing dataset without significant resource investments.
Key Features of AI Labeling Tools
Effective AI labeling tools share certain key features that enhance their usability and effectiveness:
1. Easy-to-Use Interfaces
User-friendly interfaces ensure that even those with minimal tech experience can navigate and utilize the tool efficiently, promoting wider adoption within organizations.
2. Customization Options
Organizations can tailor AI labeling tools to fit their specific needs, allowing for customization of labels, categories, and workflows according to individual project requirements.
3. Quality Control Mechanisms
Many AI labeling tools incorporate stringent quality control features to verify the correctness of annotations, ensuring that the end output is reliable for training AI models.
4. Integration with Other Platforms
Seamless integration capabilities with existing systems streamline the data annotation process, promoting collaboration across different departments and applications.
Applications of AI Labeling Tools
The application of AI labeling tools spans across numerous industries and sectors. Below are some notable examples:
1. Healthcare
In the healthcare sector, AI labeling tools assist in annotating medical images, facilitating the training of AI models that can detect anomalies in X-rays or MRIs, ultimately aiding in early diagnosis and treatment plans.
2. Automotive
The autonomous vehicle industry relies heavily on labeled data for training self-driving algorithms. AI labeling tools can streamline the annotation of road signs, vehicles, pedestrians, and various environmental conditions.
3. Retail
Retailers use AI labeling tools to analyze customer feedback and sentiment from product reviews and social media, giving insights into consumer behavior and preferences.
4. Finance
In finance, AI labeling tools facilitate the classification of transactional data, helping in fraud detection and compliance monitoring through properly annotated financial datasets.
Choosing the Right AI Labeling Tool for Your Business
When selecting an AI labeling tool to enhance your data annotation processes, consider the following factors:
1. Budget and Costs
Evaluate the pricing model of the tool—whether it’s a subscription, pay-per-use, or one-time fee—and ensure it aligns with your budget. Consider potential savings in labor costs as well.
2. Support and Community
Access to a robust support system and an active user community can be invaluable. Look for platforms that offer tutorials, forums, and responsive customer service.
3. Trial and Evaluation
Test the tool through a trial period or a demo to assess its functionality, ease of use, and fit for your specific requirements before committing long-term.
Case Study: Implementation of AI Labeling Tools at Keylabs.ai
Keylabs.ai has been a pioneer in harnessing the power of AI labeling tools to streamline data annotation processes across multiple sectors. One notable case study involved a large-scale healthcare project where accuracy in medical image annotation was critical.
By utilizing Keylabs.ai's sophisticated AI labeling tools, the project team was able to:
- Annotate over 100,000 medical images within a tight deadline.
- Achieve annotation accuracy rates exceeding 95% through advanced quality control measures.
- Reduce project costs by 40% compared to traditional manual annotation methods.
This success is a testament to how effectively implemented AI labeling tools can transform data annotation tasks into efficient, reliable, and cost-effective processes.
Future Trends in AI Labeling Tools
As technology continues to advance, several trends are emerging within the realm of AI labeling tools that promise to shape the future of data annotation:
1. Increased Automation
With the continuous development of AI and machine learning, future labeling tools will likely become even more automated, requiring minimal human intervention while achieving higher accuracy rates.
2. Deeper Integration of AI
Tools will increasingly incorporate advanced AI algorithms that can learn from user feedback and improve labeling capabilities over time, adapting to different projects and industries.
3. Enhanced Collaboration Features
Future tools may focus on enhancing collaboration features, allowing teams to work together seamlessly on labeling projects in real-time, regardless of their physical location.
4. Privacy and Security Focus
As data privacy concerns grow, there will be a significant emphasis on employing secure label processing and storage methods, ensuring adherence to regulations while maintaining data integrity.
Conclusion
In today's data-driven landscape, the importance of AI labeling tools cannot be overstated. Their ability to improve efficiency, accuracy, and cost-effectiveness makes them an indispensable asset for any organization looking to leverage machine learning and AI technologies.
As businesses like Keylabs.ai continue to innovate in the field of data annotation, the future looks promising for organizations eager to harness the power of their datasets through these cutting-edge tools. By choosing the right AI labeling tool and understanding its features and applications, companies can propel their AI initiatives to new heights.