Labeling Tools for Machine Learning: A Comprehensive Guide
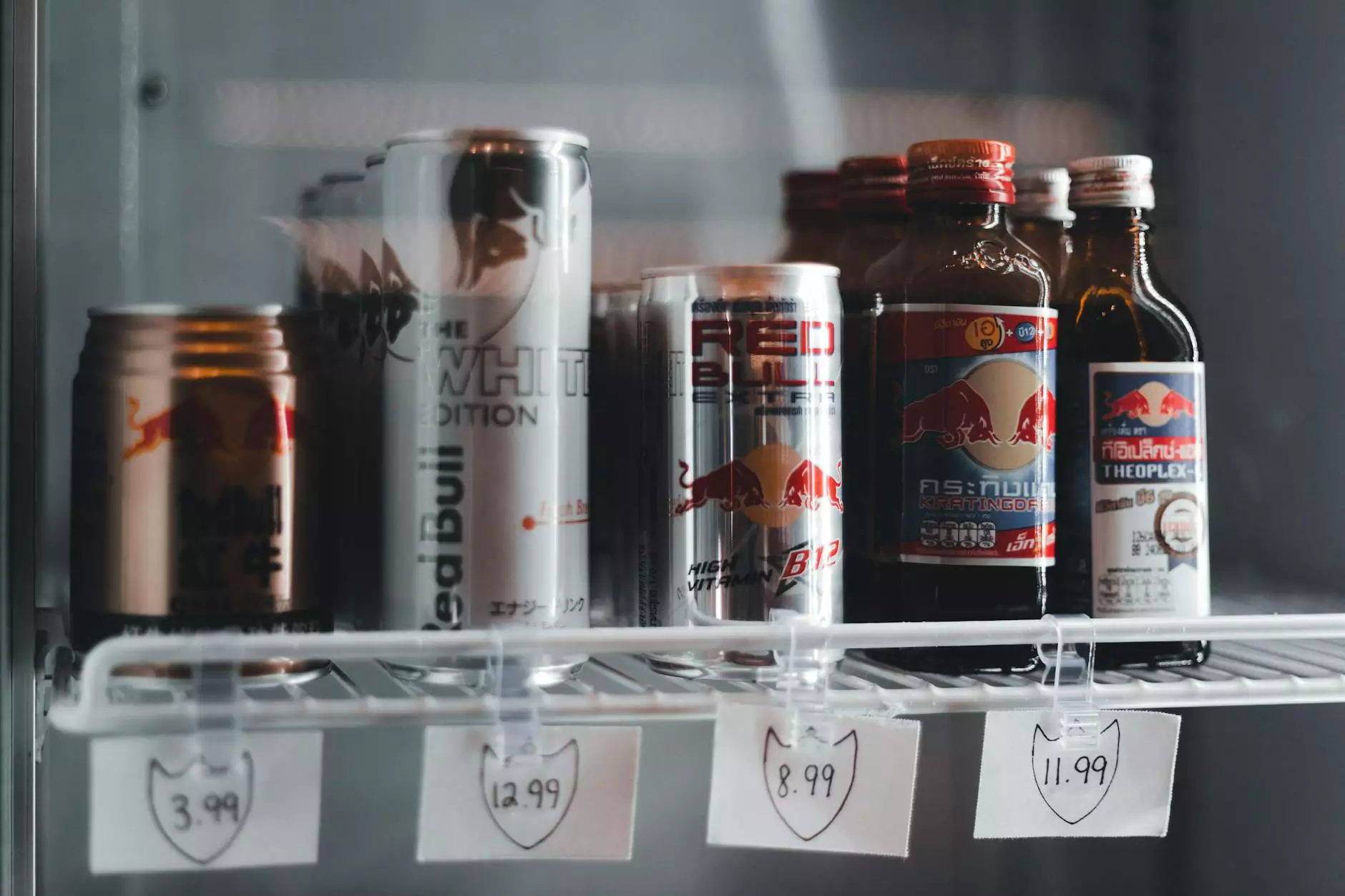
In today's rapidly evolving technological landscape, machine learning has emerged as a pivotal frontier, driving innovation across various sectors. At the heart of this transformation lies data, particularly how it is prepared and annotated for machine learning applications. The reliability and accuracy of machine learning models heavily depend on the quality of data provided, which raises the question: what are the best labeling tools for machine learning? In this article, we will explore the essential aspects of labeling tools, their significance, and how platforms like Keylabs.ai are revolutionizing the data annotation landscape.
The Importance of Data Annotation
Data annotation is the process of labeling data to make it understandable for machine learning algorithms. This step is crucial, as it directly impacts the performance of models trained to make predictions or classifications. High-quality labeled data ensures that these models can learn effectively, minimizing errors and enhancing predictive power.
Imagine teaching a child to recognize different animals. If you only show them pictures without names, they will struggle to understand. However, by labeling each image (e.g., "dog", "cat", "elephant"), you provide a structured learning experience. Similarly, in the world of machine learning, labeled datasets serve as a foundation for successful training.
Types of Data Annotation
There are several types of data annotation, each suited for different applications. Here is a brief overview:
- Image Annotation: Labeling images to recognize objects, which is widely used in computer vision tasks.
- Video Annotation: Marking frames in videos to track movements, often used in surveillance and autonomous driving.
- Text Annotation: This includes labeling parts of speech or entities within a text, essential for natural language processing (NLP).
- Audio Annotation: Labeling sounds or speech in audio files, crucial for speech recognition tasks.
Common Challenges in Data Annotation
While data annotation is essential, it is not without challenges. Here are some common hurdles faced by organizations:
- Scalability: As the demand for labeled data grows, scaling the annotation process can be difficult.
- Consistency: Ensuring that different annotators apply the same criteria can lead to discrepancies.
- Quality Assurance: Maintaining high-quality standards is essential; poor labeling can lead to ineffective machine learning models.
- Cost: High-quality annotation services can be expensive, especially for large datasets.
Labeling Tools: A Game Changer for Machine Learning
To meet the challenges of data annotation, organizations require robust labeling tools for machine learning. These tools not only improve efficiency but also enhance the overall quality of the labeled data. Here are some key features to look for in labeling tools:
1. User-Friendly Interface
The effectiveness of a labeling tool largely depends on its usability. A user-friendly interface allows annotators to quickly and accurately label data, reducing the training time for new team members.
2. Collaboration Features
In many cases, data annotation is a team effort. Collaboration features, such as real-time editing and project management tools, can facilitate communication and keep everyone aligned, ensuring that annotations are consistent.
3. Customization Options
Every project has unique requirements. The ability to customize the labeling criteria or workflow can drastically improve the efficiency of the annotation process. Look for tools that can accommodate specific needs without compromising performance.
4. Quality Control Mechanisms
Tools that offer built-in quality control features can help maintain high standards. Options like reviewer feedback, consensus checking, and automated validation processes ensure that the labeled data is of superior quality.
5. Support for Multiple Data Types
Comprehensive labeling tools should support various data types, including images, text, audio, and video. This versatility enables organizations to streamline workflows and manage diverse projects within a single platform.
Exploring Keylabs.ai: Your Go-To Data Annotation Platform
Among the myriad of labeling tools, Keylabs.ai stands out as a pioneering data annotation platform that addresses the challenges discussed earlier. With a strong focus on quality and usability, Keylabs.ai offers features that make it an ideal choice for organizations aiming to enhance their machine learning projects.
Key Features of Keylabs.ai
Keylabs.ai integrates several key features designed to streamline the data annotation process:
- Intuitive User Interface: Easy navigation ensures that annotators can focus on their tasks without excessive training.
- Real-Time Collaboration: Annotators can work together seamlessly, sharing insights and maintaining consistency across projects.
- AI-Assisted Annotation: Incorporating AI into the annotation process accelerates the workflow while maintaining high-quality output.
- Custom Workflows: Clients can tailor workflows to suit specific project needs, enhancing efficiency based on their unique datasets.
- Advanced Quality Control: Robust quality control features that help verify annotation accuracy before finalizing datasets.
The Future of Data Annotation in Machine Learning
The landscape of machine learning is constantly changing, and with it, the tools and techniques used for data annotation. As labeling tools for machine learning evolve, we can expect several trends that will shape the future:
1. Increased Automation
With advances in AI and machine learning, we are likely to see more automated tools that can assist or even perform the data annotation process. This will significantly reduce the time and cost associated with manual labeling.
2. Enhanced Integration with ML Models
Future labeling tools will more likely offer seamless integration with machine learning models, allowing for a more direct feedback loop. This will enable automatic updates and adjustments based on model performance.
3. Greater Focus on Quality and Standards
As the demand for high-quality labeled data increases, we can expect standards to emerge in data annotation practices, focusing on providing consistent and reliable outputs.
Conclusion
In conclusion, the success of machine learning initiatives heavily relies on quality data annotation, underscoring the critical role of labeling tools for machine learning. Platforms like Keylabs.ai provide the functionality and features necessary to meet the evolving needs of data annotation.
By investing in the right labeling tools, organizations can overcome the challenges of data annotation, ensuring that their machine learning models are built on a solid foundation. The journey ahead in the domain of machine learning is bright, and with reliable data annotation tools, we are poised to unlock unprecedented potential in AI development.